The Next Generation of AI: A Universal Framework for Sequential Decision Problems
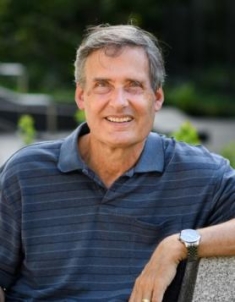
Warren B. Powell, PhD
Chief Innovation Officer
Optimal Dynamics Professor Emeritus
Princeton University Executive-in-Residence
Rutgers Business School
Abstract: Sequential decision problems are an almost universal problem class, spanning engineering, the sciences, transportation, supply chain management, health, energy, e-commerce, and finance. In contrast with deterministic optimization, sequential decision problems are studied by a number of communities using names such as dynamic programming, stochastic programming, optimal control, reinforcement learning, stochastic search, simulation-optimization and multi-armed bandit problems. These fields use eight different notational systems to describe a wide range of overlapping methods, motivated by different applications. I will present a universal modeling framework that can be used for any sequential decision problem in the presence of different sources of uncertainty. I use a “model first” strategy that optimizes over policies for making decisions. I will present four (meta)classes of policies that are the foundation of any solution approach that has ever been proposed for a sequential problem, either in the research literature or used in practice (including policies that have not been invented yet). A major theme of the talk will involve building a bridge between classical machine learning and optimizing policies for sequential decision problems. The use of parameterized deterministic approximations is easily one of the most overlooked tools in stochastic optimization. I am also going to make the case that many (most?) deterministic optimization problems are actually policies for solving sequential decision problems (three of the four classes of policies have imbedded optimization problems that are usually solved using deterministic methods). This will fundamentally change how we view deterministic optimization. I will close by making the case for teaching sequential decision analytics to a broad audience, including both graduate students in domainoriented fields, as well as undergraduates in both methodological and domain-oriented departments
Biography: Warren B. Powell is Professor Emeritus at Princeton University, where he taught for 39 years, and is currently the Chief Innovation Officer at Optimal Dynamics. He was the founder and director of CASTLE Lab, which focused on stochastic optimization with applications to freight transportation, energy systems, health, e-commerce, finance and the laboratory sciences, supported by over $50 million in funding from government and industry. He has pioneered a new universal framework that can be used to model any sequential decision problem, including the identification of four classes of policies that spans every possible method for making decisions. This is documented in his latest book with John Wiley: Reinforcement Learning and Stochastic Optimization: A unified framework for sequential decisions. He published over 250 papers, five books, and produced over 60 graduate students and post-docs. He is the 2021 recipient of the Robert Herman Lifetime Achievement Award from the Society for Transportation Science and Logistics, the 2022 Saul Gass Expository Writing Award. He is a fellow of Informs, and the recipient of numerous other awards.